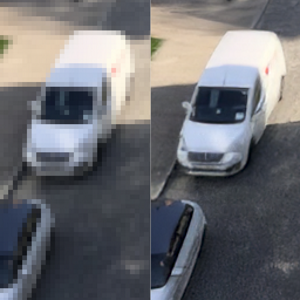
running NNSuperResolution
This weekend we rebuilt our development environment from the ground up. This includes updating graphics driver, CUDA, cuDNN and recompiling multiple layers of software to now support the latest line of graphics cards from NVIDIA, the RTX30xx series (RTX3070, RTX3080 and RTX3090). In more technical terms it means we are now supporting compute capabilities 8.0 and 8.6 (please see https://en.wikipedia.org/wiki/CUDA). The newly built version variants of NNSuperResolution are available from the Downloads page as usual. If you do indeed own a RTX30xx card, be sure to look for the versions compiled against CUDA11.2.
Cheers,
David